Data Analytics
The Hidden Dangers of Bias in Healthcare Data Analytics
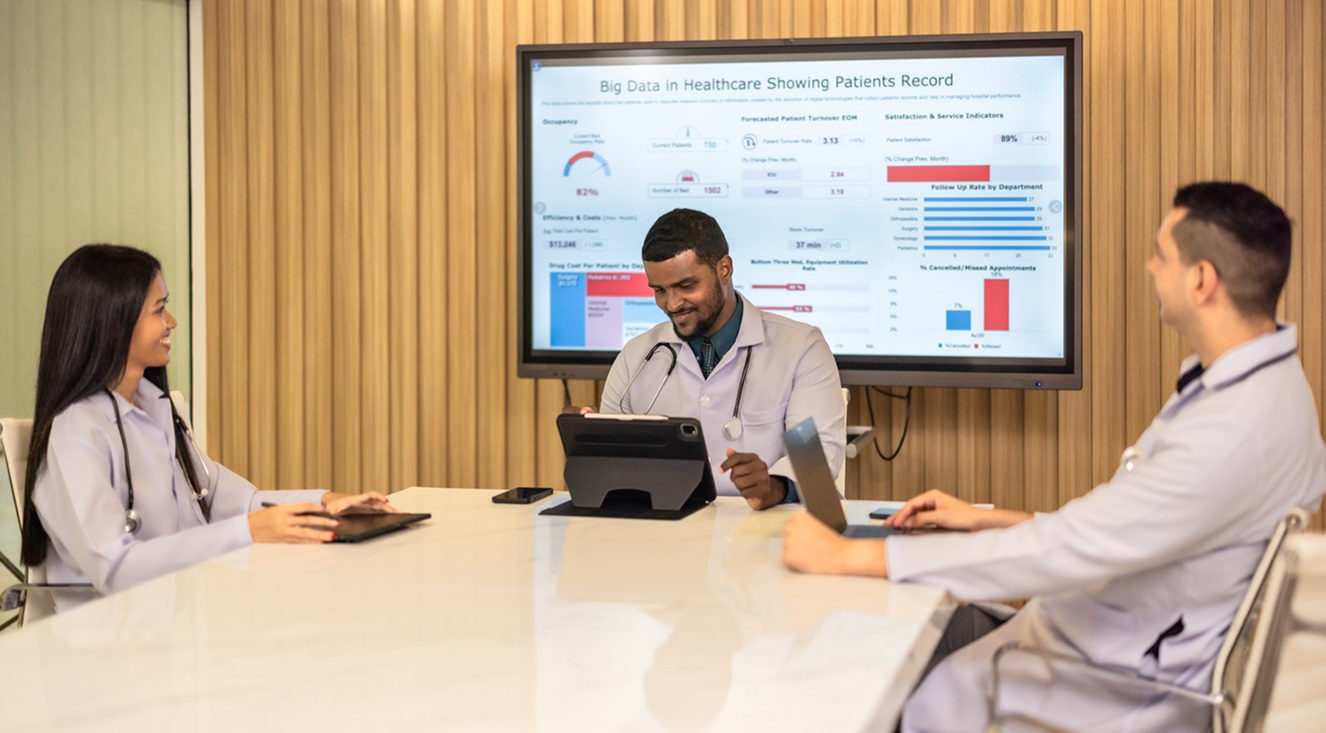
Introduction
Data analytics has become a powerful tool in modern healthcare, enabling predictive diagnostics, personalized treatments, and operational efficiency. However, the effectiveness of healthcare analytics relies heavily on the quality and integrity of the data being used. Bias in healthcare data analytics poses serious risks, leading to inaccurate predictions, inequitable treatment plans, and systemic discrimination against certain patient populations.
As artificial intelligence (AI) and machine learning (ML) become increasingly integrated into healthcare decision-making, understanding and addressing bias in data analytics is more critical than ever. This article explores the hidden dangers of bias in healthcare data, its impact on patient outcomes, and strategies to mitigate these risks.
Understanding Bias in Healthcare Data Analytics
What is Bias in Healthcare Data?
Bias in healthcare data occurs when systematic errors or distortions in data collection, analysis, or interpretation lead to unfair or inaccurate conclusions. These biases can arise from:
- Historical inequalities in healthcare access
- Incomplete or unrepresentative datasets
- Algorithmic biases in AI-driven healthcare models
- Human bias in medical decision-making
Types of Bias in Healthcare Analytics
- Selection Bias
- Occurs when the dataset does not represent the broader patient population.
- Example: Clinical trials historically underrepresent women and racial minorities, leading to treatment guidelines that may not be effective for these groups.
- Sampling Bias
- Arises when certain demographics are overrepresented or underrepresented in healthcare studies.
- Example: AI models trained on data from urban hospitals may not accurately predict health outcomes for rural populations.
- Algorithmic Bias
- AI and ML models can inherit biases from historical data, reinforcing disparities in care.
- Example: A predictive algorithm for heart disease may be less accurate for women if the training data is predominantly male patients.
- Measurement Bias
- Happens when data collection methods favor certain outcomes or populations.
- Example: Pulse oximeters have been found to overestimate oxygen levels in darker skin tones, leading to misdiagnoses.
- Confirmation Bias
- When pre-existing beliefs influence data interpretation, reinforcing stereotypes.
- Example: AI models predicting opioid abuse may disproportionately flag low-income patients or racial minorities, leading to biased risk assessments.
The Real-World Impact of Bias in Healthcare Data
1. Disparities in Disease Diagnosis and Treatment
- AI models trained on biased data can misdiagnose or undertreat certain populations.
- Example: Black patients are less likely to receive adequate pain medication because AI models have been trained on biased pain assessment data.
2. Worsening Health Inequalities
- Bias in healthcare analytics exacerbates existing disparities, particularly among marginalized communities.
- Example: AI-driven cancer detection tools trained primarily on light-skinned individuals may fail to detect skin cancer in darker-skinned patients.
3. Flawed Predictive Analytics in Risk Assessment
- Predictive models used in hospital resource allocation, insurance approvals, and treatment prioritization can unfairly disadvantage certain groups.
- Example: AI-based sepsis prediction models may under-diagnose cases in women, as most training data has been derived from male patients.
4. Ethical and Legal Consequences
- Biased AI decisions can lead to medical malpractice claims, regulatory scrutiny, and loss of patient trust.
- Healthcare institutions that use biased AI models risk violating anti-discrimination laws and ethical medical standards.
How to Mitigate Bias in Healthcare Data Analytics
1. Ensure Diverse and Representative Data
- Healthcare datasets must include diverse populations across race, gender, geography, and socioeconomic status.
- Incentivize diverse clinical trials to ensure that AI models are trained on representative data.
2. Implement Bias Auditing for AI Models
- Conduct regular audits of AI and ML models to identify potential biases.
- Use explainable AI (XAI) methods to make algorithmic decision-making more transparent.
3. Address Bias in Data Collection
- Use standardized, unbiased data collection methods to improve accuracy.
- Example: AI models should be trained on multi-site hospital data to ensure they work across different demographics.
4. Integrate Human Oversight in AI-Driven Decisions
- Healthcare providers should use AI as a decision-support tool, not a replacement for clinical judgment.
- Regularly cross-check AI-generated recommendations with expert medical review.
5. Promote Ethical AI Development in Healthcare
- Develop guidelines for responsible AI usage in healthcare that prioritize fairness, transparency, and accountability.
- Collaborate with ethicists, policymakers, and patient advocacy groups to ensure equitable AI implementation.
The Future of Ethical Healthcare Analytics
The integration of AI in healthcare is inevitable, but it must be equitable, transparent, and fair. Future advancements in bias detection algorithms, AI fairness frameworks, and ethical data governance will play a crucial role in mitigating bias in healthcare analytics.
- Regulatory bodies (such as the FDA and WHO) are working on AI ethics guidelines to ensure fairness in medical AI.
- Next-generation AI models will incorporate bias correction mechanisms to reduce disparities in diagnosis and treatment.
- Patient-centric AI systems will prioritize individualized treatment over one-size-fits-all models, reducing bias-related harm.
By addressing bias in healthcare data analytics today, we can build a more inclusive, effective, and fair healthcare system for the future.
Conclusion
Bias in healthcare data analytics is a hidden but serious threat to medical decision-making, patient care, and health equity. AI-driven healthcare solutions must be designed, tested, and implemented responsibly to avoid reinforcing systemic biases.
Healthcare institutions, AI developers, and policymakers must collaborate to create unbiased, ethical, and patient-centered AI models. By ensuring diverse data representation, conducting AI bias audits, and integrating human oversight, we can prevent biased healthcare analytics from harming patients and worsening health disparities.
AI has the potential to revolutionize medicine—but only if it is built on principles of fairness, transparency, and accountability. The future of healthcare depends on getting AI right—for everyone.
Data Analytics
The Role of Big Data in Early Cancer Detection and Treatment
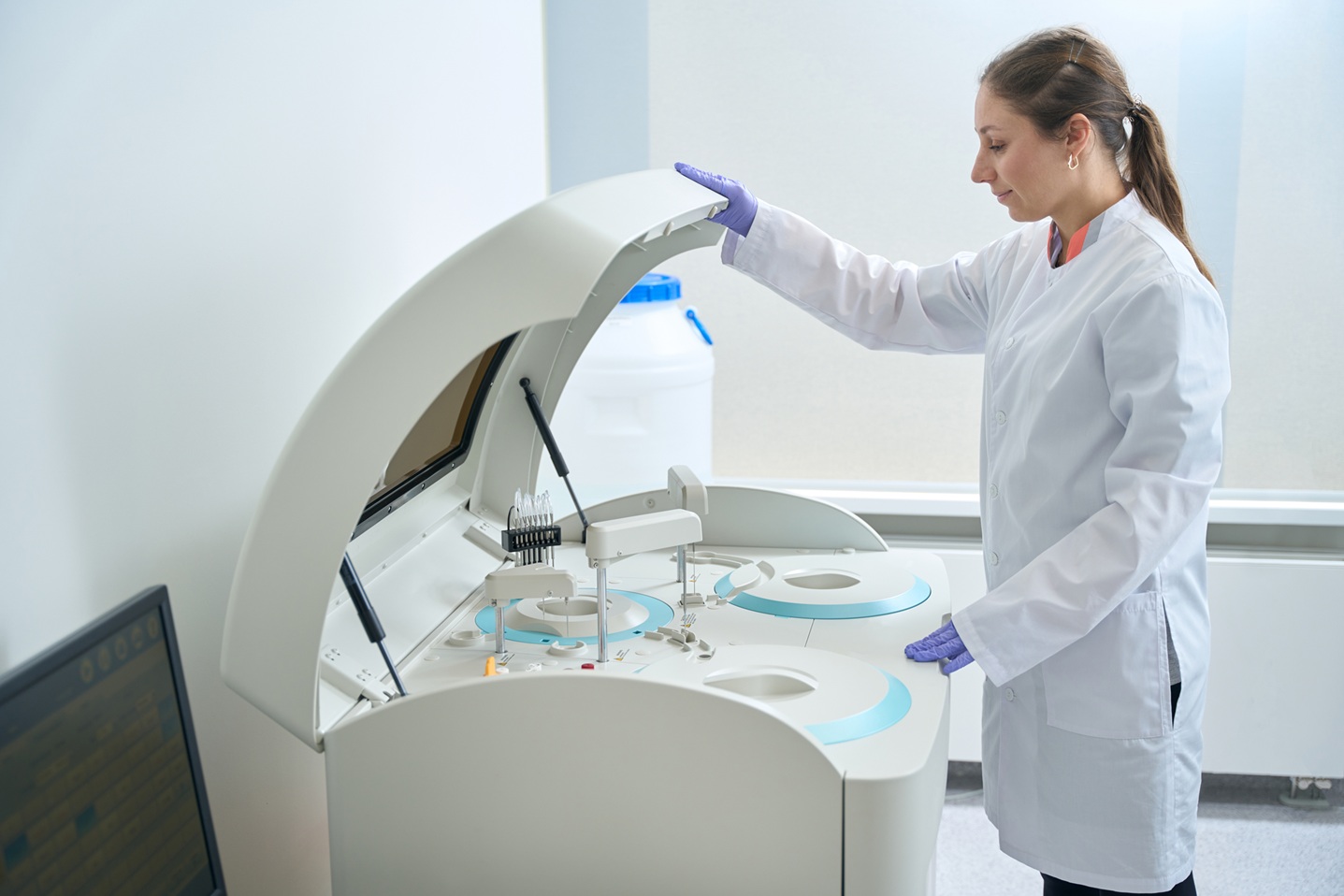
Cancer remains one of the leading causes of death worldwide, with millions of new cases diagnosed each year. Early detection and personalized treatment are crucial in improving survival rates, but traditional diagnostic methods and treatment plans have limitations. Big Data is revolutionizing oncology, offering new ways to detect cancer earlier, enhance treatment precision, and improve patient outcomes.
With the integration of artificial intelligence (AI), machine learning, and real-time analytics, Big Data is transforming how we understand, diagnose, and treat cancer. This article explores the significant role Big Data plays in the early detection and treatment of cancer, along with its challenges and future potential.
1. How Big Data Aids in Early Cancer Detection
A. AI-Powered Imaging and Screening
Medical imaging, such as mammograms, CT scans, and MRIs, is essential for detecting cancerous tumors. However, human interpretation has limitations, often leading to delayed or missed diagnoses. Big Data and AI-powered algorithms are improving imaging accuracy and detecting cancer in its earliest stages.
- Machine learning models can analyze thousands of medical scans in seconds, identifying patterns that radiologists might miss.
- Google’s DeepMind and IBM Watson have developed AI systems that detect breast cancer and lung cancer with higher accuracy than human experts.
- AI-driven histopathology analysis enhances the detection of abnormal cell structures in biopsies, reducing false positives and negatives.
B. Predictive Analytics for Cancer Risk Assessment
Big Data allows for the analysis of vast amounts of genetic, lifestyle, and environmental factors to predict an individual’s risk of developing cancer.
- Genomic sequencing combined with AI can identify genetic mutations associated with cancer, enabling early intervention.
- Predictive modeling analyzes patient history and medical records to flag high-risk individuals, allowing for more frequent screenings and preventive measures.
- Wearable technology and biosensors track real-time biomarkers, detecting early warning signs of diseases like skin cancer, colorectal cancer, and leukemia.
C. Population Health Data and Early Detection Strategies
Big Data aggregates information from millions of patients worldwide, enabling researchers to identify trends and early indicators of cancer.
- Epidemiological data helps researchers identify cancer patterns based on demographics, location, and lifestyle habits.
- Real-time data collection from hospitals, genetic testing labs, and electronic health records (EHRs) ensures early warning systems for emerging cancer risks.
2. Big Data’s Role in Personalized Cancer Treatment
Once a patient is diagnosed with cancer, Big Data enhances treatment options by tailoring therapies to the individual’s genetic profile, tumor characteristics, and response patterns.
A. Precision Medicine and Genomic Data Analysis
Cancer treatment is shifting away from a one-size-fits-all approach to personalized medicine, thanks to Big Data.
- Genomic sequencing allows doctors to match patients with the most effective targeted therapies.
- AI analyzes massive datasets to recommend customized drug combinations based on a patient’s specific cancer type and mutation profile.
- The Cancer Genome Atlas (TCGA) provides extensive genomic data, helping researchers develop more precise treatment plans.
B. Real-Time Monitoring and Adaptive Therapy
Big Data allows continuous tracking of cancer patients, ensuring timely adjustments in treatment.
- AI-driven treatment algorithms analyze real-time patient responses to chemotherapy, radiation, and immunotherapy, optimizing dosages and minimizing side effects.
- Liquid biopsies, powered by Big Data, detect circulating tumor DNA (ctDNA) in blood samples, allowing for early detection of treatment resistance.
- Wearable health monitors track patient vitals, ensuring that oncologists receive real-time alerts on adverse reactions or disease progression.
C. Drug Discovery and Clinical Trials Acceleration
The process of developing new cancer drugs typically takes years, but Big Data is speeding up the discovery and testing phases.
- AI analyzes millions of drug interactions, identifying potential new cancer treatments faster than traditional research methods.
- Machine learning models predict how patients will respond to experimental treatments, improving the efficiency of clinical trials.
- Real-world patient data helps pharmaceutical companies design better drugs by understanding which treatments work best for specific populations.
3. Challenges and Ethical Concerns in Using Big Data for Cancer Care
A. Data Privacy and Security Risks
- Cancer patients’ genomic and medical data are highly sensitive, requiring robust cybersecurity measures to protect against data breaches.
- Strict regulations, such as HIPAA (Health Insurance Portability and Accountability Act) and GDPR (General Data Protection Regulation), ensure patient confidentiality and ethical data usage.
B. Bias and Inequality in Data Sets
- Bias in medical data can lead to inaccurate predictions, especially for underrepresented populations in cancer research.
- Researchers must ensure that AI models are trained on diverse datasets to improve treatment accessibility for all demographics.
C. Integration with Traditional Healthcare Systems
- Many hospitals still use outdated electronic health record (EHR) systems, making it difficult to integrate Big Data solutions.
- Seamless data sharing between hospitals, research centers, and pharmaceutical companies is needed to maximize the impact of Big Data on cancer treatment.
4. The Future of Big Data in Cancer Care
The integration of AI, cloud computing, and real-time analytics will continue to shape the future of cancer detection and treatment. Emerging trends include:
A. AI-Powered Virtual Oncologists
- AI-driven virtual assistants may support oncologists by analyzing massive datasets in seconds, offering treatment recommendations based on real-time data.
B. Predictive Analytics for Cancer Prevention
- AI will advance predictive cancer models, allowing for customized lifestyle recommendations to prevent cancer before it develops.
C. Blockchain for Secure Medical Data Sharing
- Blockchain technology can enhance secure data sharing between hospitals, ensuring faster and more accurate diagnoses.
D. Real-Time Global Cancer Surveillance
- AI-powered surveillance will help track cancer trends worldwide, providing early warnings for emerging cancer hotspots and guiding public health policies.
Conclusion
Big Data is transforming the fight against cancer by improving early detection, enhancing personalized treatment, and accelerating drug development. AI-driven predictive analytics, real-time monitoring, and genomic insights are making cancer treatment more precise, effective, and accessible.
However, challenges related to data privacy, bias, and healthcare integration must be addressed to fully unlock the potential of Big Data in oncology. With continued advancements in machine learning, cloud computing, and digital health platforms, Big Data will play an even greater role in saving lives and redefining cancer care in the years to come.
The future of cancer treatment is not just about curing the disease—it’s about preventing it before it even starts.
Data Analytics
The Power of Real-Time Data Analytics in Emergency Medicine
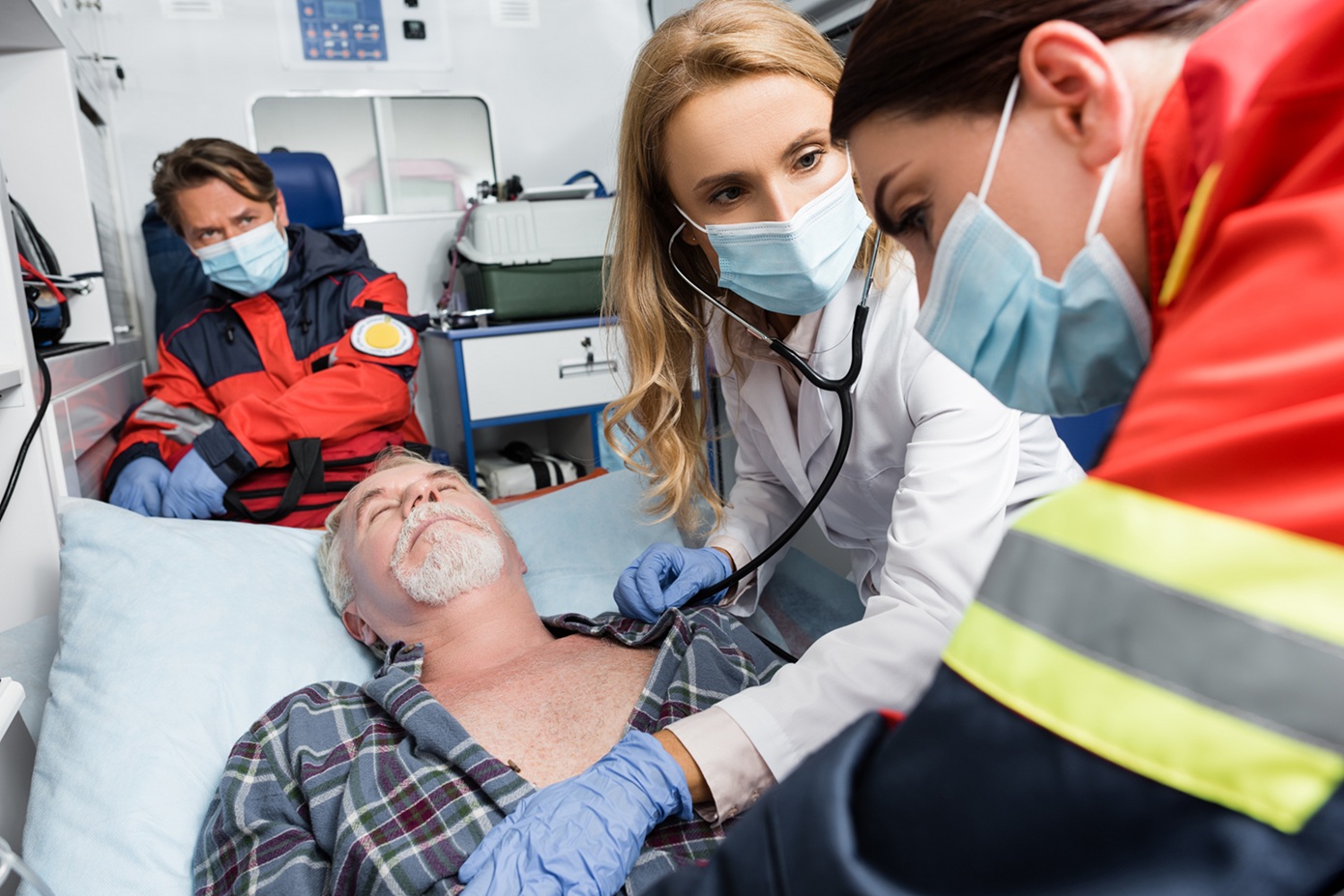
The Power of Real-Time Data Analytics in Emergency Medicine
Emergency medicine is a high-stakes field where every second counts. Quick, informed decision-making can mean the difference between life and death. Traditional medical practices often rely on delayed data processing, but the advent of real-time data analytics is revolutionizing emergency care. By leveraging instant data insights, healthcare providers can improve patient outcomes, optimize resource allocation, and enhance overall hospital efficiency.
The Role of Real-Time Data in Emergency Medicine
Real-time data analytics involves the continuous collection, processing, and interpretation of patient data as it happens. This technology utilizes Artificial Intelligence (AI), the Internet of Things (IoT), and predictive analytics to provide medical professionals with instant insights. Emergency rooms (ERs), ambulances, and trauma centers now rely on real-time analytics to enhance decision-making, reduce errors, and improve patient survival rates.
Key Benefits of Real-Time Data Analytics
1. Rapid Diagnosis and Treatment
In emergency medicine, time is the most critical factor. Real-time analytics can instantly process patient vitals, lab results, and imaging scans, helping physicians diagnose conditions like heart attacks, strokes, and sepsis within minutes. For example:
- AI-powered ECG analysis can detect abnormal heart rhythms and alert cardiologists instantly.
- Automated blood test analysis can identify sepsis risk in real-time, ensuring immediate treatment.
2. Optimizing Emergency Room (ER) Workflow
Overcrowded emergency rooms often lead to long wait times and patient dissatisfaction. Real-time data systems monitor ER congestion, predict patient inflow, and allocate staff accordingly.
- Predictive analytics can estimate patient wait times and recommend bed assignments.
- AI-driven triage systems categorize patients based on urgency, ensuring critical cases receive immediate attention.
3. Improving Ambulance and Pre-Hospital Care
Emergency medical services (EMS) now use real-time GPS tracking and remote patient monitoring to optimize ambulance response times and prepare hospitals before the patient arrives.
- Paramedics can transmit live patient data (ECGs, blood pressure, oxygen levels) to hospitals, allowing ER teams to prepare in advance.
- AI-powered navigation helps ambulances avoid traffic congestion, reducing transit delays.
4. Enhancing Disaster and Mass Casualty Management
During natural disasters, pandemics, or mass casualty incidents, real-time data analytics helps coordinate emergency responses efficiently.
- Hospitals can track patient admissions, bed availability, and resource usage in real time to avoid system overload.
- AI-driven surveillance systems analyze data from multiple sources to detect outbreaks, bioterrorism threats, or epidemic patterns early.
5. Reducing Medical Errors and Enhancing Patient Safety
Real-time data minimizes human errors by cross-referencing patient history, medication records, and lab results.
- AI-powered alerts warn doctors of potential drug interactions, allergies, or contraindications.
- Continuous monitoring systems detect sudden patient deterioration, triggering immediate interventions.
Challenges and Considerations
Despite its benefits, real-time data analytics in emergency medicine faces challenges:
- Data Security and Privacy – Hospitals must ensure compliance with HIPAA and other regulations to protect sensitive patient data.
- Integration with Existing Systems – Many hospitals use outdated electronic health records (EHR) that may not be compatible with advanced analytics platforms.
- Training and Adoption – Medical staff must be trained to use real-time analytics tools effectively to maximize their potential.
The Future of Real-Time Data Analytics in Emergency Medicine
The future of emergency medicine lies in the seamless integration of AI, wearable health devices, and 5G technology.
- AI-driven chatbots may assist ER teams by analyzing symptoms before patients even arrive.
- Wearable biosensors will continuously monitor high-risk patients, sending alerts to emergency teams when abnormal readings are detected.
- Cloud-based analytics will enable hospitals worldwide to share real-time data, improving global emergency response coordination.
Conclusion
Real-time data analytics is transforming emergency medicine, providing faster diagnoses, optimizing workflows, and ultimately saving lives. As technology continues to evolve, its integration into emergency care will become even more sophisticated, ensuring healthcare providers have the right information at the right time to make life-saving decisions. The future of emergency medicine is not just about speed—it’s about intelligence, precision, and efficiency.
Data Analytics
How Blockchain Can Improve Security in Healthcare Data Analytics
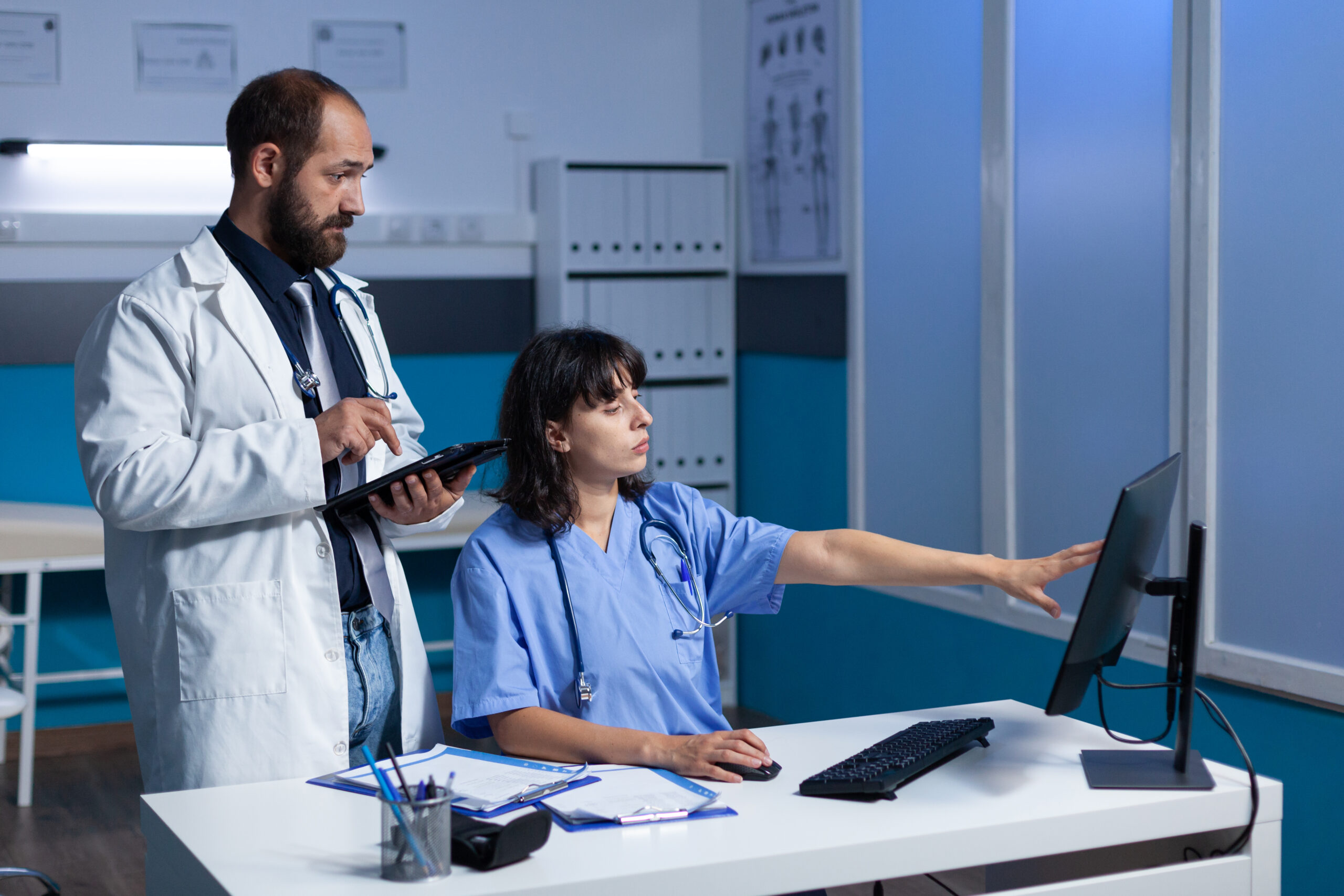
Introduction
The healthcare industry is undergoing a data revolution, with advanced analytics, artificial intelligence (AI), and machine learning transforming patient care, medical research, and hospital management. However, as healthcare data becomes more digitized, it also becomes more vulnerable to cyberattacks, data breaches, and unauthorized access.
Blockchain technology is emerging as a game-changer in healthcare data security, offering a decentralized, tamper-proof, and transparent system for storing and sharing sensitive patient information. By integrating blockchain with healthcare data analytics, institutions can enhance security, improve data integrity, and ensure compliance with global privacy regulations.
This article explores how blockchain can fortify healthcare data analytics, its real-world applications, benefits, and challenges in implementation.
1. The Growing Security Challenges in Healthcare Data Analytics
With the rise of big data and AI-driven healthcare analytics, medical organizations process enormous amounts of patient records, diagnostic data, and genomic information. However, current data management systems have significant security flaws:
🔹 Data Breaches & Cyber Threats
- Healthcare records are a prime target for cybercriminals. In 2023 alone, over 113 million patient records were compromised due to data breaches.
🔹 Lack of Data Integrity
- Centralized databases are vulnerable to unauthorized modifications, fraud, and hacking attempts, leading to false medical records or altered diagnoses.
🔹 Interoperability & Data Fragmentation
- Hospitals, insurers, and pharmaceutical companies use different data formats and incompatible EHR (Electronic Health Record) systems, leading to data silos and inefficiencies.
🔹 Privacy & Compliance Issues
- Regulations like HIPAA (U.S.), GDPR (Europe), and HITECH impose strict data security and privacy requirements, making compliance complex.
Solution: Blockchain provides a secure, decentralized infrastructure that eliminates these vulnerabilities while enhancing trust and transparency in healthcare analytics.
2. How Blockchain Strengthens Security in Healthcare Data Analytics
Blockchain technology creates a decentralized, immutable ledger, ensuring secure data storage, access, and sharing. Here’s how it enhances healthcare data analytics security:
🔹 1. Decentralized & Tamper-Proof Data Storage
Traditional healthcare data is stored in centralized servers, making it susceptible to hacking and unauthorized modifications. Blockchain eliminates single points of failure by distributing data across multiple nodes, ensuring:
✅ Immutability: Once data is recorded, it cannot be altered or deleted without network consensus.
✅ Data Integrity: Ensures authenticity and accuracy of patient records, preventing medical fraud.
🔹 Example:
- Estonia’s National Healthcare System has integrated blockchain to store citizen health records, ensuring real-time data integrity and security.
Outcome: Blockchain prevents data corruption, insider attacks, and unauthorized changes to medical records.
🔹 2. Secure & Permissioned Data Access
Blockchain allows only authorized users (doctors, hospitals, researchers, and insurers) to access patient data through smart contracts and encrypted keys.
✅ Patient-Controlled Access: Patients can grant or revoke permissions to specific healthcare providers.
✅ Zero Trust Security Model: Healthcare institutions can verify every access request without relying on a central authority.
🔹 Example:
- Medicalchain uses blockchain to allow patients to control their EHRs, granting access only to trusted doctors and researchers.
Outcome: Patients regain ownership of their data, preventing unauthorized data access and identity theft.
🔹 3. Blockchain-Powered Data Interoperability
One of the biggest challenges in healthcare analytics is the lack of seamless data exchange between hospitals, research institutions, and insurance providers. Blockchain enables:
✅ Standardized, cross-platform medical record access across multiple healthcare providers.
✅ Encrypted, real-time data sharing without the risk of manipulation.
🔹 Example:
- IBM Watson Health & Blockchain are developing a global blockchain-based medical data exchange network for interoperability between hospitals, pharmaceutical companies, and insurers.
Outcome: Blockchain eliminates data silos, reduces administrative delays, and enhances real-time patient care.
🔹 4. Fraud Prevention & Insurance Claim Security
Blockchain significantly reduces insurance fraud and false medical claims by ensuring data authenticity and transaction transparency.
✅ Immutable Claims Processing: Once a medical procedure is logged, it cannot be altered, preventing fraudulent billing.
✅ Automated Smart Contracts: Insurance claims can be auto-verified based on blockchain-validated medical data.
🔹 Example:
- Change Healthcare uses blockchain to track medical billing transactions, reducing fraudulent claims and speeding up payments.
Outcome: Blockchain ensures fraud-proof healthcare transactions, saving billions in unnecessary costs.
🔹 5. Strengthening Genomic & Clinical Research Data Security
Genomic and pharmaceutical research involve sensitive patient data, often stored in centralized databases, making them a target for cyberattacks.
✅ Encrypted DNA & Genomic Data Storage: Blockchain protects genetic information from data theft.
✅ Secure Drug Trial Data: Ensures tamper-proof documentation of clinical trials and drug efficacy reports.
🔹 Example:
- Nebula Genomics uses blockchain to securely store DNA sequencing data, allowing users to control access to their genetic information.
Outcome: Blockchain improves research transparency, prevents clinical trial fraud, and safeguards genomic privacy.
3. Key Benefits of Blockchain in Healthcare Data Analytics
✅ 100% Data Integrity & Security: No unauthorized changes or alterations.
✅ Improved Patient Privacy & Control: Patients manage their own medical records.
✅ Seamless Data Sharing & Interoperability: Faster and more secure access to health data.
✅ Fraud Prevention in Medical Billing & Insurance: Eliminates fraudulent claims.
✅ Faster Emergency Response: Doctors can access accurate, real-time patient data instantly.
Blockchain makes healthcare data analytics secure, efficient, and trustworthy.
4. Challenges & Barriers to Blockchain Adoption in Healthcare
Despite its potential, blockchain faces challenges in widespread healthcare adoption:
1. Scalability Issues
- Storing large medical datasets on blockchain requires high computational power and storage.
- Solution: Implement off-chain data storage with blockchain for faster processing.
2. Regulatory & Compliance Uncertainty
- Global healthcare regulations (HIPAA, GDPR) lack clear blockchain-specific guidelines.
- Solution: Governments must develop blockchain-specific healthcare regulations.
3. High Implementation Costs
- Healthcare providers need new IT infrastructure, increasing initial costs.
- Solution: Hybrid blockchain models can reduce costs by combining on-chain and off-chain storage.
4. Resistance from Traditional Healthcare Systems
- Hospitals and insurers are hesitant to replace legacy systems with blockchain-based solutions.
- Solution: Start with pilot projects and gradual blockchain integration.
Despite these challenges, blockchain adoption in healthcare is accelerating globally.
5. The Future of Blockchain in Healthcare Data Analytics
By 2030, blockchain is expected to be a standard security protocol in healthcare analytics, enabling:
🔹 AI-Blockchain Integration: Secure AI-driven healthcare predictions using encrypted blockchain data.
🔹 Global Blockchain Health Networks: Interconnected health data systems across countries.
🔹 Blockchain-Powered Personalized Medicine: Secure real-time genomic data analytics for customized treatments.
Blockchain is shaping the future of secure, decentralized, and patient-centric healthcare.
Conclusion
Blockchain is redefining security in healthcare data analytics, ensuring tamper-proof records, encrypted data sharing, and fraud prevention. Despite challenges in scalability and adoption, its ability to enhance privacy, compliance, and interoperability makes it a game-changing technology for healthcare.
As blockchain adoption grows, healthcare providers, insurers, and researchers must embrace its potential to create a more secure, transparent, and efficient healthcare ecosystem.
The future of healthcare security is blockchain-powered. Are you ready?
-
Artificial Intelligence2 months ago
Can AI Predict the Next Pandemic? AI in Epidemiology and Public Health
-
Artificial Intelligence2 months ago
How AI is Revolutionizing Medical Diagnoses: From Radiology to Pathology
-
Artificial Intelligence2 months ago
AI in Cancer Detection: How Machine Learning is Transforming Oncology
-
Healthtech2 months ago
The Future of Smart Pills: How Ingestible Sensors Can Improve Treatment Adherence
-
Artificial Intelligence2 months ago
AI in Medical Robotics: From Surgery Assistance to Patient Care
-
Healthtech2 months ago
The Ethics of AI and Data Privacy in Healthcare Technology
-
Artificial Intelligence2 months ago
AI-Powered Precision Medicine: Personalized Treatments for Better Outcomes
-
Artificial Intelligence2 months ago
Enhancing MRI and CT Scans with AI: Faster and More Accurate Diagnostics