Healthtech
How Machine Learning is Revolutionizing Drug Discovery and Development
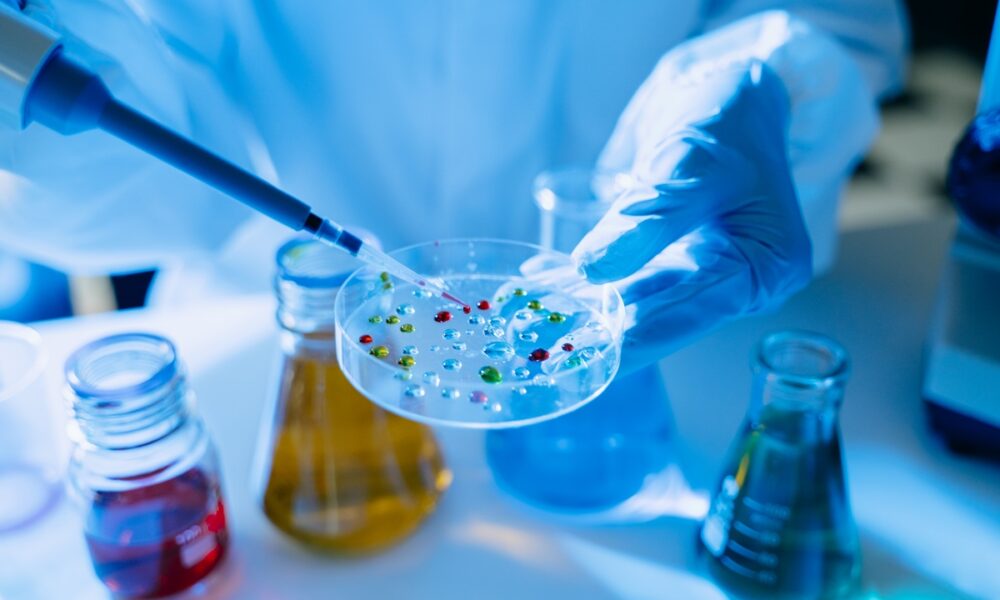
The pharmaceutical industry is undergoing a transformative shift, driven by the integration of machine learning (ML) into drug discovery and development. Traditional drug discovery methods are time-consuming, costly, and often lead to high failure rates. With the advent of ML, researchers can analyze vast datasets, predict molecular interactions, and accelerate the process of bringing new drugs to market. This article explores how machine learning is revolutionizing drug discovery and its potential impact on the future of medicine.
The Role of Machine Learning in Drug Discovery
Machine learning leverages artificial intelligence (AI) to analyze complex biological and chemical data, identifying potential drug candidates with higher accuracy and speed. Key applications of ML in drug discovery include:
1. Identifying Drug Targets and Biomarkers
- ML algorithms analyze genetic and clinical data to identify new drug targets.
- Predictive models detect biomarkers for personalized medicine, improving treatment efficacy.
2. Accelerating Drug Screening and Design
- Deep learning models predict how molecules interact with biological targets, reducing the need for extensive laboratory experiments.
- Generative adversarial networks (GANs) create novel drug compounds with optimized properties.
3. Optimizing Clinical Trials
- ML-driven analytics help identify suitable patient populations for clinical trials, reducing trial costs and time.
- Real-time monitoring of patient responses improves adaptive trial designs and increases success rates.
4. Predicting Drug Toxicity and Side Effects
- ML models analyze historical data to predict adverse effects of new compounds before human trials.
- This reduces the likelihood of late-stage failures, saving time and resources.
Benefits of Machine Learning in Drug Development
1. Speeding Up the Drug Discovery Process
- ML can analyze millions of chemical compounds in a fraction of the time required for traditional methods.
- Automated workflows enhance research productivity, enabling faster drug approvals.
2. Reducing Costs
- AI-powered simulations lower the expenses associated with trial-and-error laboratory experiments.
- Efficient resource allocation minimizes financial risks in drug development.
3. Enhancing Precision Medicine
- ML-driven insights enable the creation of personalized treatment plans tailored to individual genetic profiles.
- Predictive analytics improve patient outcomes by recommending the most effective therapies.
Challenges in Implementing Machine Learning in Drug Discovery
1. Data Quality and Availability
- ML models require vast amounts of high-quality, standardized data for accurate predictions.
- Collaboration among pharmaceutical companies and research institutions is crucial for data sharing.
2. Regulatory and Ethical Concerns
- AI-generated drug candidates must undergo rigorous testing and regulatory approval to ensure safety.
- Ethical considerations around AI-driven decision-making in healthcare must be addressed.
3. Integration with Traditional Drug Development Pipelines
- Many pharmaceutical companies still rely on conventional methodologies, requiring a shift toward AI-driven approaches.
- Workforce training and adaptation to new AI tools are necessary for seamless integration.
The Future of Machine Learning in Drug Development
As ML continues to evolve, its impact on drug discovery will expand further. Key future trends include:
- Quantum computing integration for enhanced molecular simulations.
- AI-driven robotic labs automating drug synthesis and testing.
- Collaborative AI models improving cross-industry research and data sharing.
Conclusion
Machine learning is revolutionizing drug discovery and development by accelerating research, reducing costs, and enhancing precision medicine. While challenges remain, ongoing advancements in AI, data science, and computational biology will continue to reshape the pharmaceutical industry. By embracing machine learning, drug developers can unlock new possibilities, bringing life-saving treatments to patients faster and more efficiently than ever before.